Techbio and its applications in BrainTech: an investor perspective
We have decided to dedicate a series of articles on the BrainTech market to show our investor perspective. After discussing the Brain Technology Revolution, Brain and Nutrition, BCI, Psychedelics and Sleep tech, Skills Enhancement this new article deals with the current state of Techbio and its applications in BrainTech.
Introduction
There are plenty of different definitions of what a Techbio company is. We start with the definition provided by MMC Ventures:
In this article we choose to focus on companies that want to enhance drug discovery and drug development (R&D) processes thanks to technologies, with the objective to accelerate these steps and reduce the cost for pharma and biotech companies.
Why now?
Tech tools become valuable to accelerate innovation, especially for pathology with strong unmet needs and high development cost thanks to:
- The massive amount of data generated by the Bio sector:
The digitization of the biology and biotech sector (Bio sector) is reinforced by the virtualization of samples and research findings, thanks to the power of the cloud. This key step has transformed data-sharing capabilities, changing the way data is used. The seamless exchange of vast quantities of data has strengthened collaboration and facilitated global analysis on an unprecedented scale.
Despite the exponential increase in data, bringing investigational drugs to market has not significantly improved. The industry is realizing the need to translate data into actionable insights in drug discovery. This has led to a shift in mindset, with companies adopting a data/engineering and statistical perspective to transform traditional R&D processes. Startups are mining vast datasets for valuable insights using sophisticated algorithms. This approach should be complemented by human-derived and clinical biomarker data to deepen our understanding of disease pathology. This integrated strategy holds the key to unlocking the potential of digitalization in reshaping biology and biotechnology.
Genomics data is expected to require up to 40,000 petabytes of storage each year by 2025, compared to X (fka Twitter) which requires 17 petabytes a year or all the data collected on astronomy (1,000 petabytes a year).
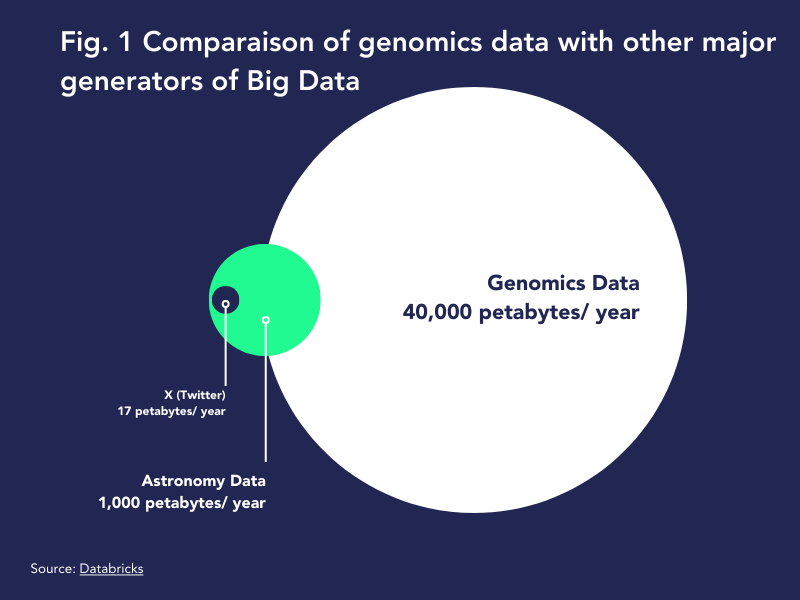
- The cost of sequencing leads to the acceleration of AI & ML
The significant reduction in sequencing time and costs in -omics disciplines has transformed biotechnology, making data much more accessible. This accessibility has diversified biological information access and created opportunities for startups to leverage these datasets. Companies like Ultima Genomics, Illumina, 10X Genomics, and Pacific Biosciences are leading advancements in sequencing technologies, aiming to bring genome sequencing costs below $100 per genome within five years (compared to $100 million in 2001) (Cf.Figure 2).
The abundance of multimodal data provides insights into complex diseases, to understand the underlying mechanisms of diseases. Lower costs mean access to quality information that was clearly impossible before. What's more, the different disciplines -omics data can be combined and synergies created to extract even more actionable information to accelerate R&D.
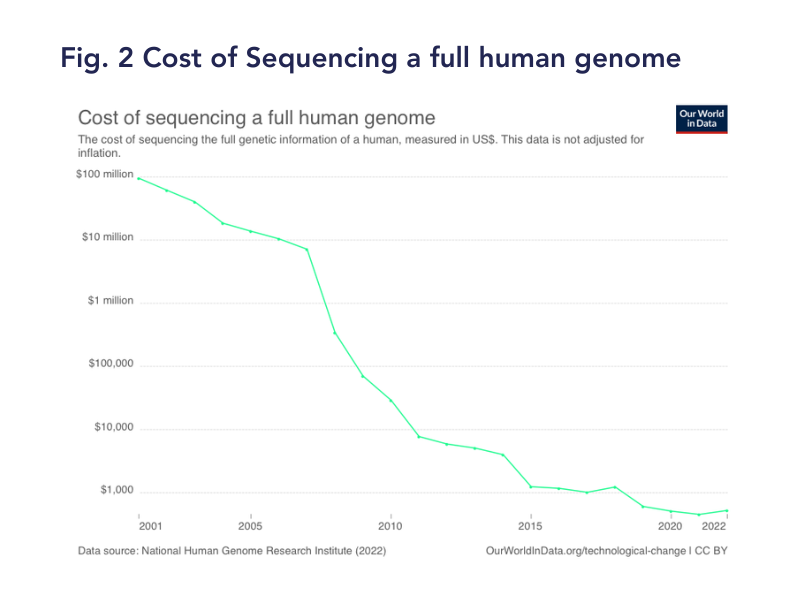
Machine learning is a very promising tool to leverage and enhance the value of these data. Moreover, generative AI seems promising for some use cases that are emerging. AI acts as a language that helps us learn the rules of biology, similar to how mathematics is the language of physics. Language models, like those trained to analyze protein structure, have revolutionized protein design, as seen with models like AlphaFold. AI also has potential in designing small molecules, RNA therapeutics, and new modalities. While AI doesn't guarantee clinical safety and efficacy, it expands the possibilities of biological discovery. Computational biology approaches are necessary for analyzing, interpreting, and extracting insights from complex datasets.
- The explosion of drug development cost
A paper published in Jama called ‘Estimated Research and Development Investment Needed to Bring a New Medicine to Market, 2009-2018’ showed the FDA approved a total of 355 new drugs and biologics. R&D expenditures were reported for 63 (18%) of these products, originating from 47 distinct companies. Factoring in the costs associated with unsuccessful trials, the base case analysis revealed a median capitalized R&D investment of $1.14 Bn and a mean investment of $1.56 Bn to bring a new drug to market. Therapeutic area-specific median estimates ranged from $0.77 Bn for central nervous system (CNS) drugs to $2.77 Bn for oncology and immunology drugs. The available data primarily pertained to smaller firms, orphan drugs, products in specific therapeutic areas, first-in-class drugs, therapeutic agents with accelerated approval, and products approved between 2014 and 2018.
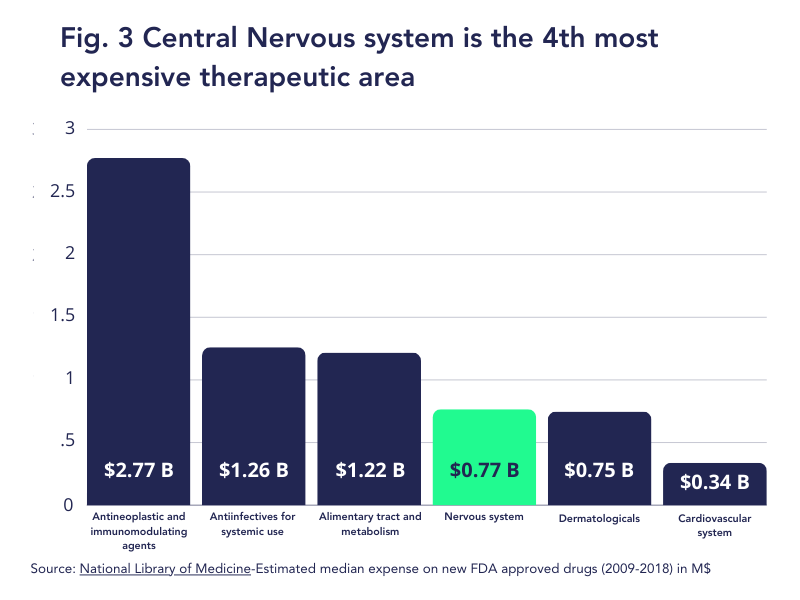
Techbio could be a solution for complex and costly pathologies
Within the Techbio Landscape, companies related to oncology have seen significant development. Indeed, in the case of oncology the technologies bring a lot of value for several reasons:
- The complexity of disease and biological mechanism: AI excels at analyzing large-scale data to identify specific mutations and molecular targets.
- High Unmet Medical Need: cancer is still a leading cause of mortality worldwide, nearly one in six deaths.
- Rich Data Sources: The field of oncology has benefited from extensive data generation through initiatives like The Cancer Genome Atlas (TCGA)
- High drug development cost: As we saw on the previous part oncology and immunology are the area where the drug development costs are the higher.
Here are some examples:
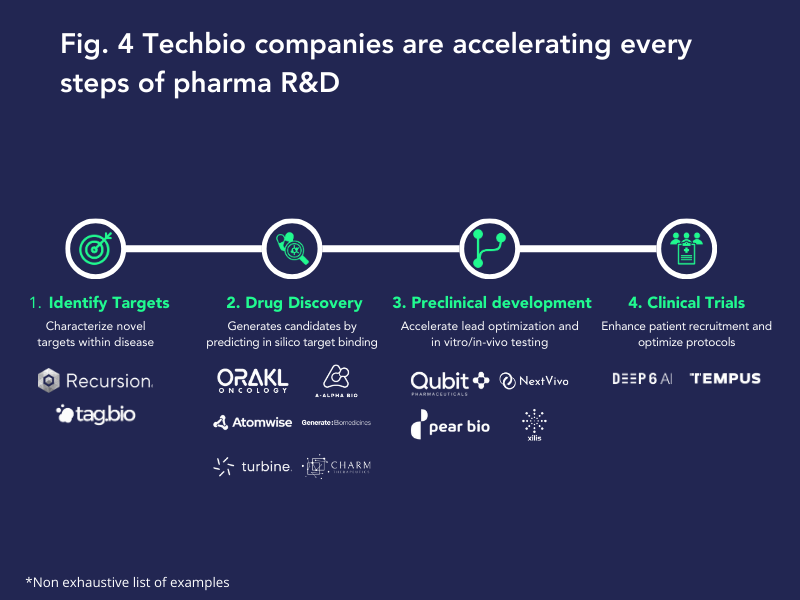
The aging of the population and the development of chronic diseases are two of the major challenges facing public health worldwide. In particular, 1.5 billion people suffer from neurological and psychiatric diseases. Newfund has witnessed a scientific revolution in the understanding of the brain, with the creation of massive amounts of data. As we have seen, TechBio companies in oncology are disrupting the R&D market. There is now momentum around CNS (Central Nervous System) companies because AI/ML algorithms can be applied to the data that is now available. So, the same criteria that paved the way for the emergence of Techbio startups in oncology apply to companies linked to the CNS:
- The complexity of disease: Disorders such as Alzheimer's, Parkinson's, and various neuropsychiatric conditions involve very complex molecular and cellular mechanisms.
- High Unmet Medical Need: Many CNS disorders lack effective treatments, and the prevalence of conditions like Alzheimer's is rising. There are over 55 million people worldwide living with dementia in 2020. This number will almost double every 20 years, reaching 78 million in 2030 and 139 million in 2050.
- Rich Data Sources: The availability of genomic and proteomic data related to CNS disorders has grown substantially. Indeed, brain bio bank have been created to accelerate research like the NeuroBioBank (USA) , the BrainHealth Databank (CA) , the biobank of functional brain images by Helsinki Brain & Mind.
- High drug development costs: In the first part, we observed that CNS drugs rank as the fourth highest sector in terms of drug development costs, making this therapeutic area one of the most expensive.
Technology to identify new targets, and model the interaction of a molecule with a target are the same regardless of the sector in which it is applied. For instance, Insitro developed a platform with a pipeline either on oncology and neuroscience. Also, Tag.bio could leverage any sort of healthcare related data, to accelerate R&D process and help their customers have more insights thanks to their data. So, technically it is possible to build a platform that is sector agnostic.
Braintech companies also have their own needs related to the particularities of diseases affecting the CNS. Indeed, these diseases are characterized by their slow progression and are composed of non-homologous groups: Techbio seems relevant to cluster the different phenotypes, for instance during clinical trials.
For Alzheimer Disease, candidate treatments seek to slow disease progression, with a modest effect, typically a cognitive decline of the order of 15-20% slower with treatment than without. Because the effect is modest, it requires many participants to be demonstrated, up to 3,000 for large phase 3 trials.One way of increasing the effect is to target participants in the trial who will experience a rapid and significant phase of cognitive decline. On these patients, the treatment effect will be stronger than on participants who would have experienced a milder decline in cognition. We can therefore evaluate the efficacy of the treatment with a smaller number of these patients, known as fast-progressors. Techbio predicts disease progression and enables us to target fast-progressors by calculating a prognostic score for each participant.
The development of all these boxes has even led to recommendations from expert : the EMA, followed by the FDA, have issued clear recommendations on the use of "prognostic scores" in clinical trials.
Another example is brain toxicity of compounds, no relevant cellular models exist today to assess the brain toxicity of a molecule. The consequence is because of many brain toxicities of molecules they failed during the passage to the clinic. Companies like Herophilus or Brainzell are leveraging biology innovation and AI to create preclinical brain models to reduce risk and accelerate the development of molecules targeting the brain.
Here are some examples:
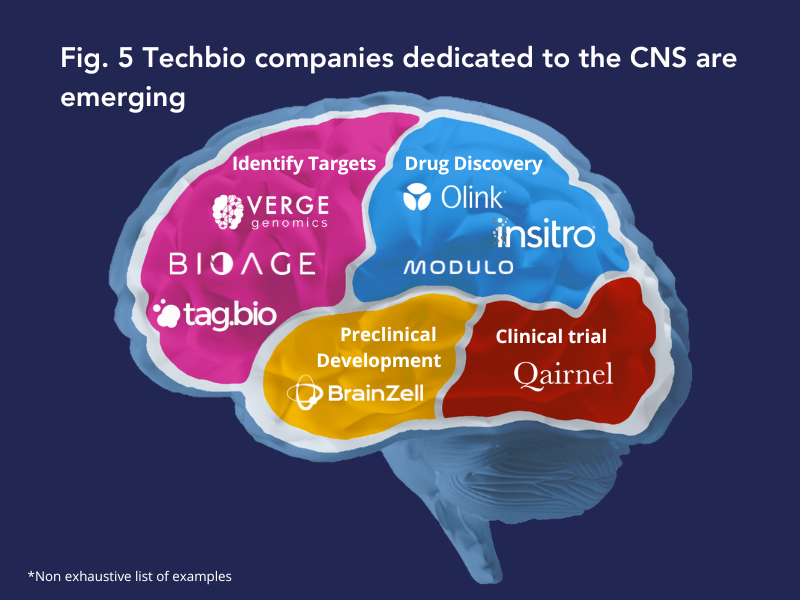
Limits
Some challenges remain for the CNS sector: drugs targeting the brain must penetrate the Blood-Brain Barrier to reach their targets, presenting a challenge beyond mere interaction with the target to ascertain if they can effectively reach and affect it.
The CNS sector presents a technical complexity because diseases are really heterogeneous. It is partly because beyond the genetic impact, the environmental impact is very strong on this type of pathology. This makes target identification even more complicated. And so, without a clear target it is even more difficult to find a compound of interest.
Conclusion
Progress in biology today gives us the ability to gather a massive amount of biodata at lower costs, which are readily available and digitalized. Advances in AI, ML, and computer science enable the processing and analysis of these vast quantities of data.
R&D costs continue to rise, especially for diseases involving complex biological mechanisms, such as those related to the brain. This has led to a significant need for Techbio solutions to address the unmet needs of brain diseases and thus accelerate the delivery of innovative new therapies to patients.
There is a very promising ecosystem surrounding Techbio for Newfund's Heka fund. Indeed, after addressing the significant needs of the oncology sector, the CNS sector will increasingly have dedicated solutions for patients with no effective treatments available, even though some challenges remain to be addressed.
We are excited to see what will come next and if you are building a Techbio company related to the brain or the CNS, feel free to reach out: constant@newfundcap.com.
“Although there are several examples of useful applications of AI/ML applications in drug discovery for CNS disorders, we have only just begun to understand the potential of these technologies for discovering effective new therapeutics.”
Harnessing the Power of Artificial Intelligence To Boost Drug Discovery by The Icahn School of Medicine at Mount Sinai